Demystifying the Technology That Powers Creativity and Automation
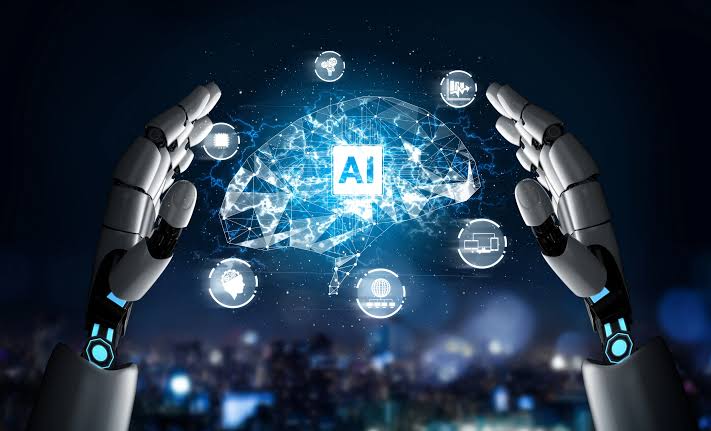
Generative-AI is revolutionizing industries by enabling machines to create content—be it text, images, music, or even code—that closely mimics human creativity. This groundbreaking technology is reshaping everything from content creation to design and beyond. But how does it work? In this beginner’s guide, we’ll break down the mechanisms, key technologies, and applications of GenerativeAI in an accessible and straightforward manner.
What is Generative AI?
Generative AI refers to a subset of artificial intelligence that focuses on generating new, original content based on input data. Unlike traditional Artificial Intelligence, which classifies or predicts, GenerativeAI creates.
For instance, it can:
- Write essays, poems, and news articles.
- Generate lifelike images and videos.
- Compose music or soundtracks.
- Design graphics or produce animations.
Generative AI models are trained on vast amounts of data, learning patterns and structures to produce unique outputs.
How Generative AI Works
1. Training Data
GenerativeAI relies on massive datasets. These datasets consist of text, images, audio, or other types of content.
- Example: A model like ChatGPT is trained on text from books, articles, and websites.
2. Machine Learning Algorithms
At the core of generative AI are machine learning algorithms. These algorithms learn from data during the training phase and generate content during the inference phase.
3. Neural Networks
Generative-AI uses deep neural networks, particularly:
- GANs (Generative Adversarial Networks): Two neural networks compete with each other, producing increasingly realistic outputs.
- Transformers: Models like GPT (Generative Pre-trained Transformer) leverage transformers for processing sequential data, such as text or time-series information.
4. Key Processes
- Encoding: The input data is converted into a numerical format that the model understands.
- Learning: The model identifies patterns, relationships, and structures in the data.
- Generation: Based on its learning, the model generates new data.
Technologies Behind Generative AI
1. GANs (Generative Adversarial Networks)
- Two networks: A generator creates content, and a discriminator evaluates it.
- They work together to produce realistic outputs, like deepfake images or synthesized voices.
2. Transformers
- Transformers power language models like GPT, which excel at understanding and generating human-like text.
- Example: ChatGPT generates coherent responses based on prompts.
3. Diffusion Models
- Used in AI art tools like DALL-E or MidJourney.
- These models generate images by iteratively refining random noise into meaningful visuals.
4. Natural Language Processing (NLP)
- Enables models to understand and generate human language.
- Example: Summarizing articles, translating languages, or generating stories.
Applications of Generative Artificial Intelligence
1. Content Creation
Generative AI streamlines writing, graphic design, and video editing.
- Example: AI writing tools like Jasper.ai draft articles or marketing copy.
2. Art and Design
AI generates stunning artwork, logos, and animations.
- Example: DALL-E creates unique images based on text descriptions.
3. Gaming
Game developers use Artificial Intelligence to design characters, worlds, and storylines.
- Example: Procedural generation of game levels.
4. Healthcare
AI creates synthetic medical data for research or generates personalized treatment plans.
5. Music and Audio
Generative AI composes original music or remixes existing tracks.
- Example: AI tools like AIVA compose soundtracks for films.
6. Education
Artificial Intelligence generates personalized study materials or interactive learning content.
Advantages of Generative AI
- Efficiency: Automates time-consuming creative tasks.
- Personalization: Creates tailored outputs for individual users.
- Scalability: Produces large volumes of content quickly.
- Innovation: Unlocks new forms of creativity and experimentation.
Challenges of Generative AI
- Ethics: Risk of misuse, such as creating deepfakes or plagiarized content.
- Quality Control: Outputs can sometimes be inaccurate or inappropriate.
- Bias: AI models may reflect biases present in their training data.
- Energy Consumption: Training large models demands significant computational resources.
The Future of Generative AI
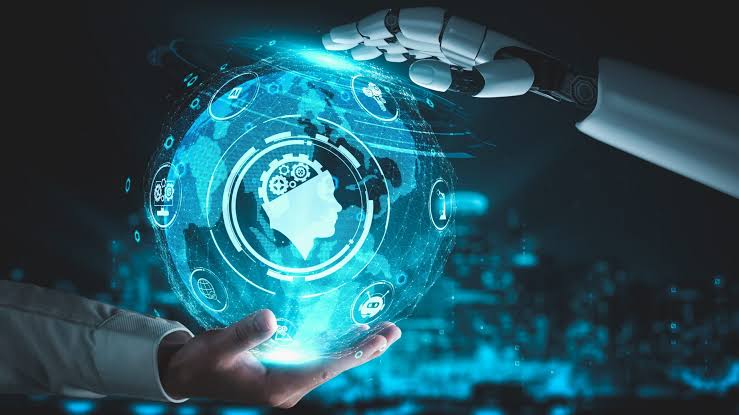
Generative AI is set to redefine creativity and problem-solving across industries. Innovations in natural language understanding, image synthesis, and multimodal models (combining text, audio, and visuals) will unlock even greater possibilities. However, with this growth comes the responsibility to address ethical and social challenges.
Generative AI is not just a tool—it’s a paradigm shift in how we think about creativity and automation. By understanding how it works and exploring its applications, we can harness its potential to innovate while navigating its challenges responsibly. Whether you’re a content creator, developer, or business leader, generative AI offers a transformative opportunity to reimagine the future.
Ready to explore the creative frontier of AI? The journey begins now.
Free Artificial Intelligence Course
Welcome to UGTWorld’s newest segment – A Complete Artificial Intelligence Free Course designed to empower learners with in-depth knowledge of AI, from fundamentals to advanced applications. This course is your gateway to understanding and mastering the dynamic world of Artificial Intelligence, with a special focus on cutting-edge fields like Generative AI and Imprinting AI.